Discover Hidden Patterns in Data
Introduction
Have you ever wondered how your favorite apps seem to anticipate what you need or how streaming services suggest movies you didn’t even know you wanted to watch? 🤔 These are all examples of hidden patterns in data at work! Hidden patterns are the secrets behind the scenes that help us make sense of the vast amounts of information swirling around us every day. Whether you're a teacher guiding your students through the maze of digital information or a student trying to make sense of your assignments, understanding these patterns can transform the way you interact with technology and the world.
Imagine walking into a classroom where every resource is perfectly organized, every app you use knows your preferences, and every problem you encounter has a clear, logical solution. Sounds like a dream, right? But it's not! By uncovering hidden patterns in data, we can create more efficient learning environments, develop smarter tools, and solve everyday problems with ease. 🌟
But what exactly are hidden patterns, and why should we care about them? Let's dive into the fascinating world of data patterns, explore how they shape our interactions, and discover how you can harness their power to overcome common challenges in education and beyond.
Whether you're a teacher looking to enhance your teaching methods or a student striving to excel in your studies, understanding hidden patterns in data can equip you with the skills to navigate the complexities of the digital age. So, let’s embark on this journey together and unlock the mysteries that data holds!
What Are Hidden Patterns?
Hidden patterns are regularities or structures in data that aren’t immediately obvious. They’re like the underlying framework that holds all the pieces together, making sense of seemingly random information. In the context of informatics, recognizing these patterns is crucial for tasks like data analysis, problem-solving, and developing intelligent systems.
Recognizing Patterns in Everyday Life
Think about how you recognize faces. Even in a crowd, you can spot your friend’s face among many others. This ability to discern familiar patterns is rooted in our brain's pattern recognition skills. Similarly, computers can be trained to recognize patterns in data, enabling them to perform tasks like image recognition, speech processing, and even predictive analytics.
💡 Insight: Pattern recognition is a fundamental aspect of both human cognition and computer science. By understanding how patterns work, we can better design systems that complement our natural abilities.
Patterns in Data
In data science, patterns can take many forms. They can be trends over time, relationships between variables, or recurring sequences. For example:
- Trends: An increase in online shopping during holidays.
- Correlations: A relationship between study time and exam scores.
- Sequences: Patterns in website navigation paths.
Recognizing these patterns allows us to make informed decisions, forecast future events, and solve complex problems.
📘 Tip: Start by looking for simple patterns in small datasets before moving on to more complex analyses. This foundational understanding will make tackling larger datasets more manageable.
Types of Patterns
Patterns can be classified into various types, each serving a different purpose in data analysis:
- Statistical Patterns: These involve numerical data and include trends, correlations, and distributions.
- Structural Patterns: These relate to the organization or relationship within data, such as hierarchical structures or network connections.
- Behavioral Patterns: These identify actions or behaviors over time, like user interactions on a website.
Understanding the type of pattern you’re dealing with is the first step in effectively analyzing and applying it.
✍️ Example: Imagine you’re tracking the attendance in your computer science classes over a semester. You notice that attendance drops slightly every Wednesday. By identifying this pattern, you might investigate whether there's a scheduling conflict or perhaps introduce a mid-week incentive to boost attendance. This simple observation can lead to actionable solutions that improve the learning environment.
Key Takeaways
- Hidden patterns are underlying structures in data that help make sense of complex information.
- Pattern recognition is essential for tasks such as data analysis, problem-solving, and developing intelligent systems.
- Different types of patterns (statistical, structural, behavioral) serve various purposes in data analysis.
- Identifying patterns can lead to informed decisions, forecasting, and effective problem-solving.
🔍 Fun Fact: The concept of pattern recognition dates back to ancient times when humans first started identifying regularities in nature, such as the changing seasons or animal behaviors.
Try This!
Quiz Question:
Which of the following is an example of a behavioral pattern?
A) The distribution of ages in a classroom.
B) The correlation between study hours and exam scores.
C) User login times on a website.
D) The hierarchical structure of a company's organizational chart.
Think about it and choose the correct option!
Empower Digital Minds Through Bebras
1,400 Schools
Enable every school in Armenia to participate in Bebras, transforming informatics education from a subject into an exciting journey of discovery.
380,000 Students
Give every student the chance to develop crucial computational thinking skills through Bebras challenges, preparing them for success in our digital world.
Help us bring the exciting world of computational thinking to every Armenian school through the Bebras Competition. Your support doesn't just fund a contest - it ignites curiosity in informatics and builds problem-solving skills that last a lifetime.
I Want to Donate Now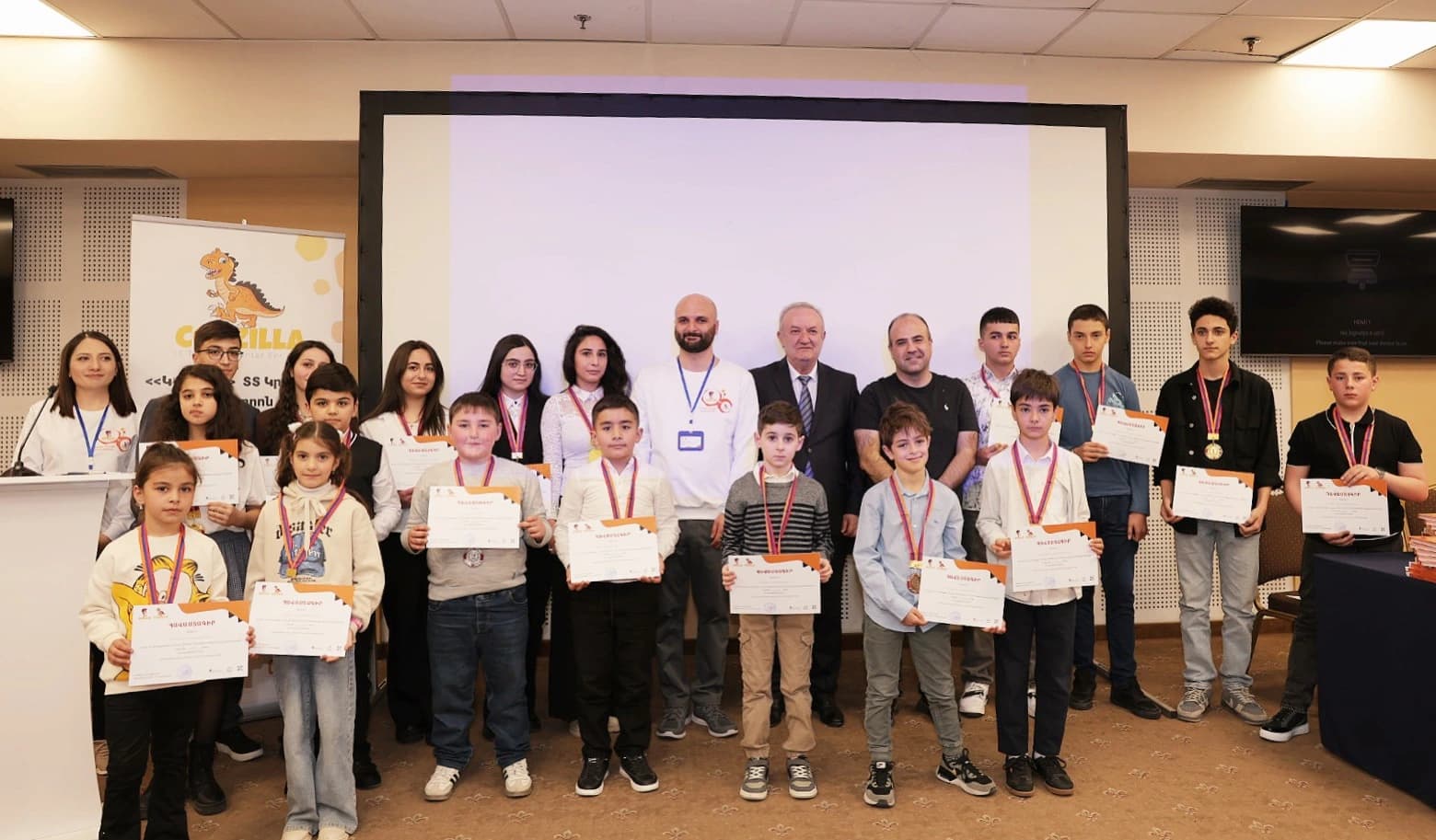
Types of Data Patterns
Understanding the different types of data patterns is essential for uncovering the hidden insights within your data. Each type provides a unique lens through which we can analyze and interpret information, leading to a more comprehensive understanding of the underlying phenomena.
Statistical Patterns
Statistical patterns deal with numerical data and often involve analyzing trends, correlations, and distributions. These patterns help us identify how variables interact and change over time.
Trends
Trends represent the general direction in which data is moving over a period. They can be upwards, downwards, or stable. For instance, tracking the number of students excelling in programming over several semesters can reveal whether teaching methods are becoming more effective.
📘 Tip: Use line graphs to visualize trends over time. This makes it easier to spot increases, decreases, or consistent patterns.
Correlations
Correlations indicate a relationship between two or more variables. A positive correlation means that as one variable increases, so does the other. Conversely, a negative correlation means that as one variable increases, the other decreases. Understanding correlations helps in predicting how changes in one aspect might affect another.
💡 Insight: Correlation does not imply causation. Just because two variables are correlated does not mean that one causes the other. It’s important to investigate further to determine the nature of the relationship.
Distributions
Distributions describe how values of a variable are spread or dispersed. They provide insights into the range, frequency, and variability of data. Common types of distributions include normal, skewed, and uniform distributions.
✍️ Example:
Imagine analyzing the distribution of test scores in a class. If most students score around the average with few high and low outliers, the distribution is normal. However, if many students score low with a few high outliers, the distribution is skewed. Understanding this helps teachers tailor their teaching strategies to address areas where students may be struggling.
Structural Patterns
Structural patterns focus on the organization and relationship within data. These patterns help in understanding how different components interact and form a cohesive whole.
Hierarchical Structures
Hierarchical structures arrange data in a ranked or ordered manner, often resembling a tree. This is common in organizational charts, file directories, and taxonomies in biology.
📘 Tip: Use tree diagrams to visualize hierarchical structures. They provide a clear view of the relationships and levels within your data.
Network Connections
Network connections illustrate how entities are linked or connected. This is prevalent in social networks, computer networks, and transportation systems.
💡 Insight: Analyzing network connections can reveal key nodes or hubs that play a critical role in the flow of information or resources.
✍️ Example:
Consider a school's library system organized in a hierarchical structure with categories like Fiction, Non-Fiction, Science, and Literature. Within each category, books are further organized by author or genre. Understanding this hierarchy helps both students and teachers locate resources efficiently.
Behavioral Patterns
Behavioral patterns identify actions or behaviors over time. These patterns are crucial for understanding how entities interact and evolve, such as user behavior on a website or studying habits of students.
Time-Based Behaviors
Time-based behaviors track how actions change over time. This can include daily routines, usage spikes during certain periods, or evolving preferences.
📘 Tip: Use time series analysis to study how behaviors change over time and identify recurring patterns or anomalies.
Sequential Behaviors
Sequential behaviors involve actions performed in a specific order. These patterns are common in processes, workflows, and learning sequences.
Empower Digital Minds Through Bebras
1,400 Schools
Enable every school in Armenia to participate in Bebras, transforming informatics education from a subject into an exciting journey of discovery.
380,000 Students
Give every student the chance to develop crucial computational thinking skills through Bebras challenges, preparing them for success in our digital world.
Help us bring the exciting world of computational thinking to every Armenian school through the Bebras Competition. Your support doesn't just fund a contest - it ignites curiosity in informatics and builds problem-solving skills that last a lifetime.
I Want to Donate Now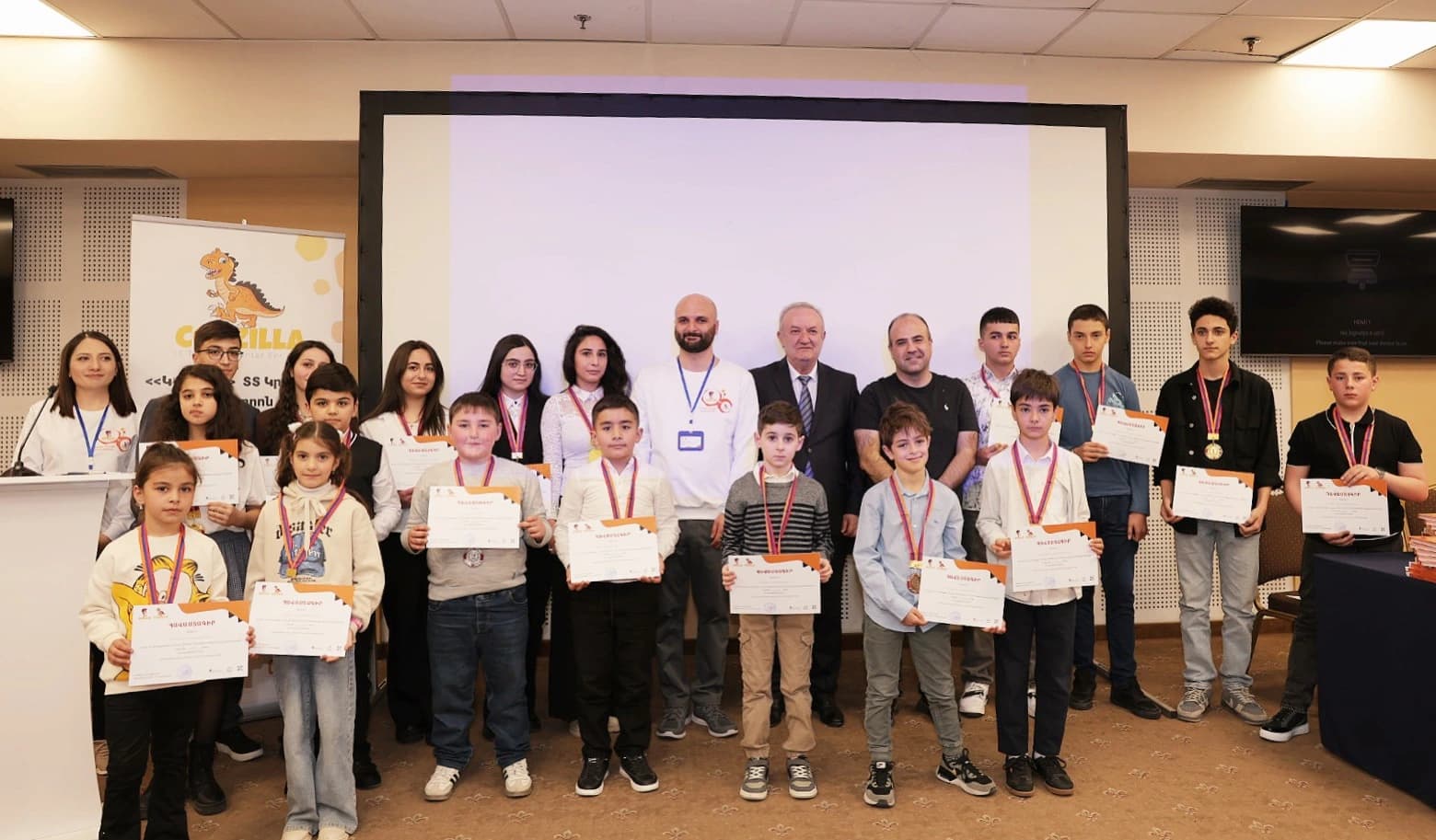
💡 Insight: Understanding sequential behaviors can help in designing more effective processes and predicting future actions based on past sequences.
✍️ Example:
In a classroom setting, you might observe that students tend to start their homework immediately after school, take a short break, and then continue working. Recognizing this sequence can help in structuring homework assignments to align with their natural workflow, potentially improving productivity and focus.
Key Takeaways
- Statistical patterns involve numerical data analysis, including trends, correlations, and distributions.
- Structural patterns focus on the organization and relationships within data, such as hierarchical structures and network connections.
- Behavioral patterns identify actions or behaviors over time, encompassing time-based and sequential behaviors.
- Each type of pattern provides a unique perspective, enhancing our ability to analyze and interpret data effectively.
🔍 Fun Fact: The study of patterns in data is a cornerstone of many scientific disciplines, including biology, economics, and computer science, demonstrating its universal importance.
Try This!
Self-Reflection Prompt:
Think about a project or subject you’re currently working on. Can you identify any statistical, structural, or behavioral patterns within it? How might recognizing these patterns help you improve your approach or outcomes?
Techniques to Uncover Hidden Patterns
Discovering hidden patterns in data isn't magic—it's a systematic process that involves various techniques and tools. By mastering these methods, you can transform raw data into meaningful insights that drive informed decisions and innovative solutions.
Data Collection and Preparation
Before patterns can be uncovered, data must be collected and prepared. This involves gathering relevant information and ensuring it’s clean, organized, and ready for analysis.
Gathering Data
Data can be collected from numerous sources, such as surveys, experiments, databases, or online platforms. The quality and relevance of your data significantly impact the patterns you can identify.
📘 Tip: Always define your objectives clearly before collecting data. Knowing what you’re looking for helps in selecting the right sources and types of data.
Cleaning Data
Data cleaning involves removing inaccuracies, filling in missing values, and standardizing formats. Clean data ensures that the patterns you uncover are accurate and reliable.
💡 Insight: Even a small amount of dirty data—like missing values or duplicates—can skew your analysis and lead to incorrect conclusions. Investing time in data cleaning pays off in the accuracy of your findings.
✍️ Example:
Suppose you’re analyzing student attendance records. You might find that some entries are missing dates or have incorrect names. By cleaning the data, you ensure that your pattern analysis, such as trends in attendance over the semester, is based on accurate information.
Exploratory Data Analysis (EDA)
Exploratory Data Analysis is the initial step in analyzing data, where you visualize and summarize key characteristics. EDA helps in identifying patterns, anomalies, and relationships within the data.
Visualization Techniques
Visualization is a powerful tool for uncovering patterns. Common visualization methods include:
- Histograms: Show the distribution of a single variable.
- Scatter Plots: Display relationships between two variables.
- Box Plots: Highlight the spread and skewness of data.
- Heatmaps: Illustrate the intensity of data points across two dimensions.
📘 Tip: Choose the right visualization based on the type of pattern you’re seeking. For instance, use scatter plots for correlation analysis and histograms for distribution insights.
Descriptive Statistics
Descriptive statistics summarize the main features of a dataset quantitatively. Key measures include mean, median, mode, standard deviation, and variance.
💡 Insight: Descriptive statistics provide a quick overview of the data’s central tendency and variability, laying the groundwork for more in-depth pattern analysis.
Empower Digital Minds Through Bebras
1,400 Schools
Enable every school in Armenia to participate in Bebras, transforming informatics education from a subject into an exciting journey of discovery.
380,000 Students
Give every student the chance to develop crucial computational thinking skills through Bebras challenges, preparing them for success in our digital world.
Help us bring the exciting world of computational thinking to every Armenian school through the Bebras Competition. Your support doesn't just fund a contest - it ignites curiosity in informatics and builds problem-solving skills that last a lifetime.
I Want to Donate Now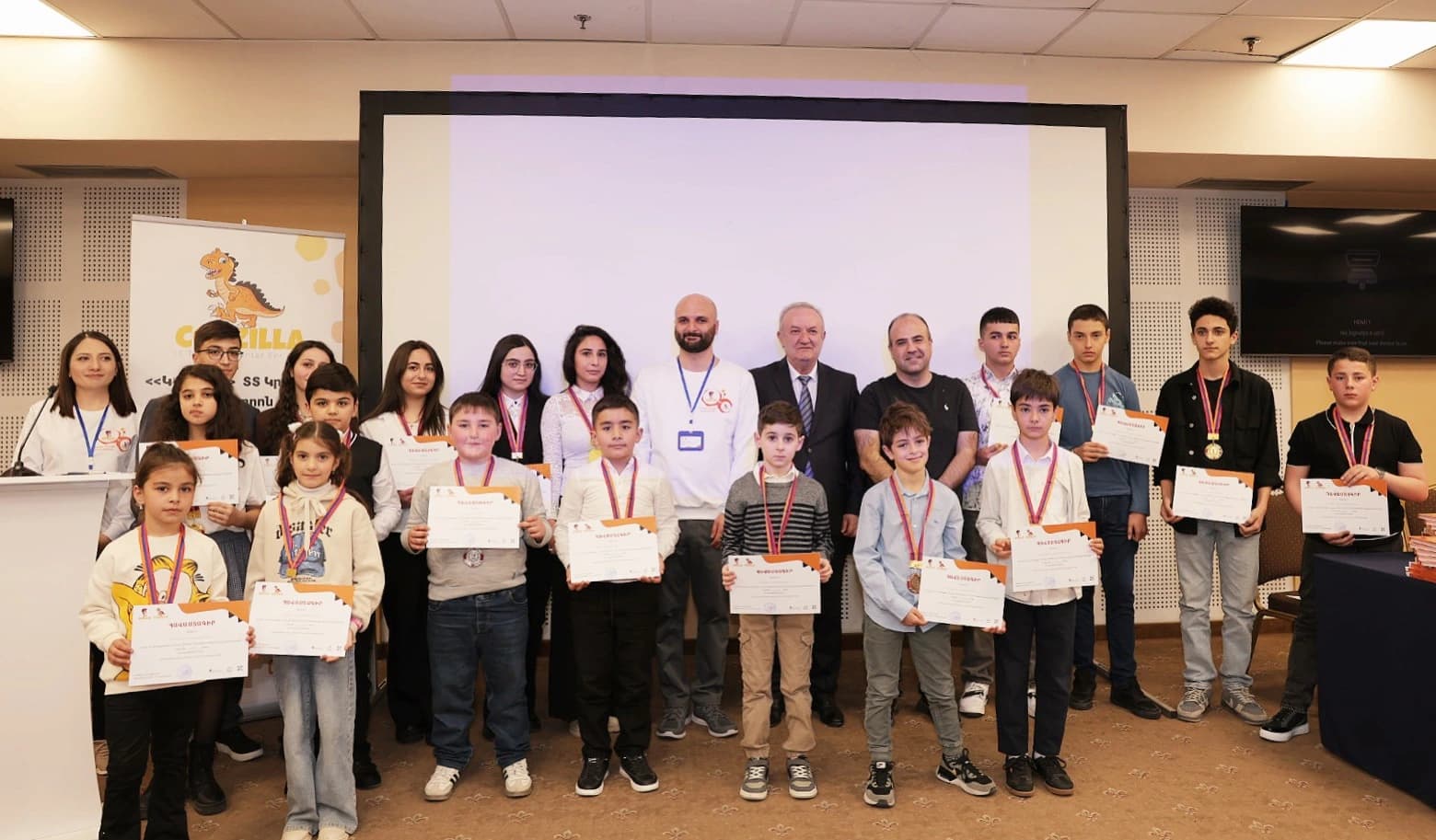
✍️ Example:
If you're studying the time students spend on homework, calculating the average (mean) time can reveal general trends. Additionally, understanding the variability (standard deviation) can show how much students’ homework times differ from the average, indicating consistency or diversity in study habits.
Machine Learning Algorithms
Machine learning algorithms are essential for identifying complex patterns that may not be apparent through traditional analysis. These algorithms can automatically learn and improve from data.
Supervised Learning
Supervised learning involves training a model on labeled data, where the outcome is known. Common supervised learning techniques include:
- Regression: Predicts continuous outcomes (e.g., predicting student grades based on study time).
- Classification: Categorizes data into predefined classes (e.g., identifying email as spam or not spam).
📘 Tip: Ensure your training data is representative of the problem you’re trying to solve to improve the accuracy of your model.
Unsupervised Learning
Unsupervised learning deals with unlabeled data, seeking to find hidden structures or groupings within the data. Common unsupervised learning techniques include:
- Clustering: Groups similar data points together (e.g., grouping students based on learning habits).
- Association: Identifies rules that describe large portions of your data (e.g., studying patterns linked to high grades).
💡 Insight: Unsupervised learning is particularly useful for exploratory analysis, where you’re unsure what patterns might exist within the data.
Reinforcement Learning
Reinforcement learning is a type of machine learning where an agent learns to make decisions by performing actions and receiving feedback. While more advanced, it’s used in areas like game playing and robotics.
✍️ Example:
Imagine you want to develop a smart tutoring system that adapts to each student’s learning pace. By using supervised learning, the system can predict which teaching methods work best based on past performance data. Over time, as more data is collected, the system becomes more accurate in personalizing education for each student.
Key Takeaways
- Data collection and preparation are foundational steps in uncovering hidden patterns, ensuring data is relevant and accurate.
- Exploratory Data Analysis (EDA) uses visualization and descriptive statistics to identify initial patterns and relationships.
- Machine learning algorithms enable the discovery of complex and non-obvious patterns through supervised, unsupervised, and reinforcement learning.
- Mastering these techniques allows for more effective data analysis, leading to insightful and actionable outcomes.
🔍 Fun Fact: The term "machine learning" was coined in 1959 by Arthur Samuel, a pioneer in the field of artificial intelligence.
Try This!
Quiz Question:
Which machine learning technique is best suited for grouping students based on their study habits without predefined categories?
A) Regression
B) Classification
C) Clustering
D) Reinforcement Learning
Take a moment to choose the best option!
Tools and Technologies for Pattern Discovery
In the digital age, numerous tools and technologies have been developed to assist in the discovery of hidden patterns in data. These tools range from simple visualization software to advanced machine learning platforms, each offering unique features to cater to different analytical needs.
Data Visualization Tools
Visualization tools transform raw data into graphical representations, making it easier to identify patterns, trends, and outliers at a glance.
Tableau
Tableau is a leading data visualization tool known for its user-friendly interface and powerful visualization capabilities. It allows users to create interactive and shareable dashboards without requiring advanced programming skills.
Empower Digital Minds Through Bebras
1,400 Schools
Enable every school in Armenia to participate in Bebras, transforming informatics education from a subject into an exciting journey of discovery.
380,000 Students
Give every student the chance to develop crucial computational thinking skills through Bebras challenges, preparing them for success in our digital world.
Help us bring the exciting world of computational thinking to every Armenian school through the Bebras Competition. Your support doesn't just fund a contest - it ignites curiosity in informatics and builds problem-solving skills that last a lifetime.
I Want to Donate Now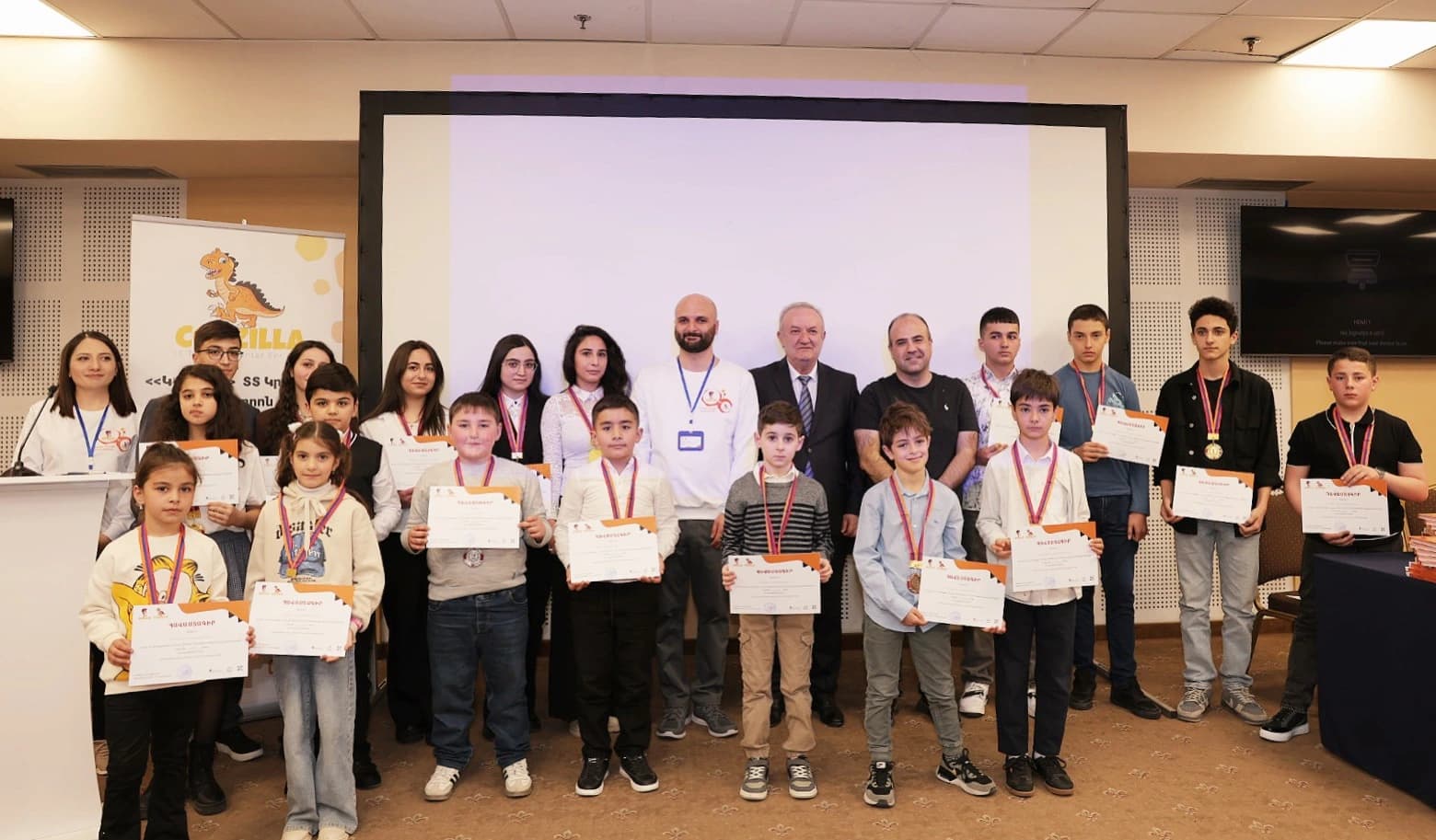
📘 Tip: Use Tableau’s drag-and-drop functionality to explore different types of visualizations and find the one that best represents your data.
Microsoft Power BI
Power BI is another popular tool that integrates seamlessly with other Microsoft products. It offers robust data visualization features and real-time data analysis, making it ideal for dynamic reporting.
💡 Insight: Power BI’s ability to connect to multiple data sources allows for comprehensive analysis across various platforms, enhancing pattern discovery.
✍️ Example:
As a teacher, you can use Power BI to visualize student performance data across different subjects and identify patterns in learning outcomes. This can help in tailoring lessons to address areas where students are struggling or excelling.
Statistical Analysis Software
Statistical analysis software provides advanced tools for performing complex data analyses, enabling deeper insights into data patterns.
R
R is a free, open-source programming language specifically designed for statistical analysis and data visualization. It offers a vast array of packages for different types of data analysis tasks.
📘 Tip: Take advantage of R’s extensive library of packages to perform specialized analyses, such as time series forecasting or spatial data analysis.
SPSS
SPSS (Statistical Package for the Social Sciences) is a widely used software for statistical analysis in social science research. It offers a user-friendly interface and robust statistical capabilities.
💡 Insight: SPSS is particularly useful for performing descriptive and inferential statistics, making it ideal for educational research and surveys.
✍️ Example:
If you’re conducting a survey to understand student satisfaction with online learning, SPSS can help you analyze the responses, identify trends, and determine which factors most significantly impact satisfaction levels.
Machine Learning Platforms
Machine learning platforms provide the necessary tools to build, train, and deploy machine learning models for pattern discovery and predictive analytics.
TensorFlow
TensorFlow, developed by Google, is an open-source platform for machine learning. It’s widely used for building neural networks and deep learning models, making it suitable for complex pattern recognition tasks.
📘 Tip: Start with TensorFlow’s pre-built models and tutorials to get a grasp of building your own machine learning algorithms.
Scikit-learn
Scikit-learn is a popular Python library that offers simple and efficient tools for data mining and data analysis. It includes a range of supervised and unsupervised learning algorithms.
💡 Insight: Scikit-learn’s straightforward API makes it an excellent choice for beginners looking to implement machine learning techniques without a steep learning curve.
✍️ Example:
Using Scikit-learn, you can create a model to predict student dropout rates based on various factors like attendance, grades, and participation. By analyzing the patterns in this data, interventions can be designed to support at-risk students.
Integrated Development Environments (IDEs)
IDEs provide comprehensive environments for writing and testing code, essential for data analysis and machine learning tasks.
Jupyter Notebook
Jupyter Notebook is an open-source web application that allows you to create and share documents containing live code, equations, visualizations, and narrative text. It’s widely used for data cleaning, visualization, and machine learning.
📘 Tip: Use Jupyter Notebook to document your data analysis process, making it easier to share insights and collaborate with others.
PyCharm
PyCharm is a powerful IDE for Python development, offering features like code completion, debugging, and version control integration. It’s particularly useful for large-scale data analysis projects.
Empower Digital Minds Through Bebras
1,400 Schools
Enable every school in Armenia to participate in Bebras, transforming informatics education from a subject into an exciting journey of discovery.
380,000 Students
Give every student the chance to develop crucial computational thinking skills through Bebras challenges, preparing them for success in our digital world.
Help us bring the exciting world of computational thinking to every Armenian school through the Bebras Competition. Your support doesn't just fund a contest - it ignites curiosity in informatics and builds problem-solving skills that last a lifetime.
I Want to Donate Now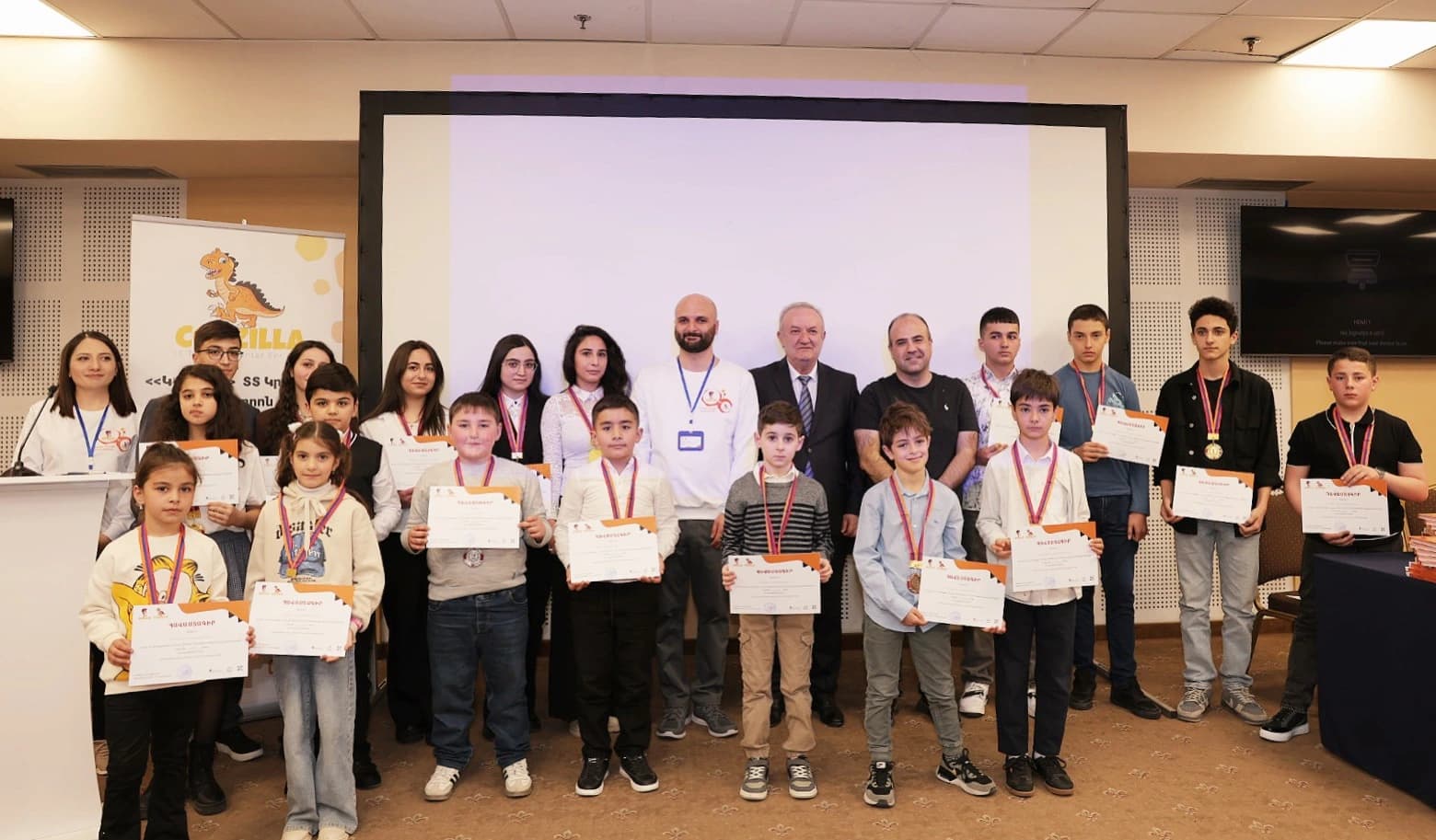
💡 Insight: PyCharm’s robust feature set helps streamline the coding process, allowing you to focus on analyzing and uncovering patterns in your data.
✍️ Example:
When working on a project to analyze student engagement in online forums, PyCharm can help you write and debug Python scripts efficiently, ensuring that your data analysis is both accurate and effective.
Key Takeaways
- Data visualization tools like Tableau and Power BI transform raw data into intuitive graphical representations, aiding in pattern recognition.
- Statistical analysis software such as R and SPSS offer advanced capabilities for performing complex data analyses and uncovering deeper insights.
- Machine learning platforms like TensorFlow and Scikit-learn provide the tools necessary to build models that can identify and predict complex data patterns.
- Integrated Development Environments (IDEs) like Jupyter Notebook and PyCharm facilitate the coding and analysis process, making data exploration more efficient and organized.
🔍 Fun Fact: The first version of R was released in 1993 by Ross Ihaka and Robert Gentleman at the University of Auckland, New Zealand.
Try This!
Self-Reflection Prompt:
Which of the tools mentioned do you feel most comfortable using, and which one would you like to learn more about? How could incorporating this tool into your teaching or learning process help you uncover new patterns in your data?
Applications of Pattern Discovery in Education
Understanding hidden patterns in data is not just an academic exercise—it has practical applications that can significantly enhance the educational experience for both teachers and students. From improving teaching methods to personalizing learning, pattern discovery can transform the classroom into a more effective and engaging environment.
Enhancing Teaching Methods
By analyzing data related to student performance, engagement, and feedback, teachers can identify patterns that inform and improve their teaching strategies.
Identifying Learning Gaps
Patterns in test scores, assignment submissions, and class participation can highlight areas where students are struggling. Recognizing these patterns allows teachers to address learning gaps proactively.
💡 Insight: Regularly tracking and analyzing performance data helps in identifying trends over time, enabling timely interventions that can prevent students from falling behind.
✍️ Example:
Suppose you notice a consistent drop in scores for a particular topic across multiple classes. This pattern suggests that the topic may not be being taught effectively, prompting you to revisit your teaching materials or methods for that subject.
Tailoring Instruction
Different students have different learning styles. By identifying patterns in how students engage with various teaching methods, teachers can tailor their instruction to better meet individual needs.
📘 Tip: Use data from class activities and assessments to experiment with different teaching approaches and see what resonates best with your students.
Personalizing Learning for Students
Personalized learning involves customizing educational experiences to meet the unique needs, skills, and interests of each student. Pattern discovery plays a crucial role in making this possible.
Adaptive Learning Systems
Adaptive learning systems use data patterns to adjust the difficulty and type of content presented to each student. This ensures that students are neither bored with material that’s too easy nor overwhelmed by content that’s too difficult.
💡 Insight: Personalized learning pathways built on data-driven patterns can increase student engagement and improve academic outcomes by catering to individual strengths and weaknesses.
Predicting Student Success
By analyzing patterns in attendance, participation, and academic performance, educators can predict which students are at risk of underperforming and implement support systems accordingly.
✍️ Example:
If data shows that students who miss more than two classes per month tend to have lower grades, you can create an early warning system to reach out to these students and provide the necessary support to help them stay on track.
Streamlining Administrative Tasks
Empower Digital Minds Through Bebras
1,400 Schools
Enable every school in Armenia to participate in Bebras, transforming informatics education from a subject into an exciting journey of discovery.
380,000 Students
Give every student the chance to develop crucial computational thinking skills through Bebras challenges, preparing them for success in our digital world.
Help us bring the exciting world of computational thinking to every Armenian school through the Bebras Competition. Your support doesn't just fund a contest - it ignites curiosity in informatics and builds problem-solving skills that last a lifetime.
I Want to Donate Now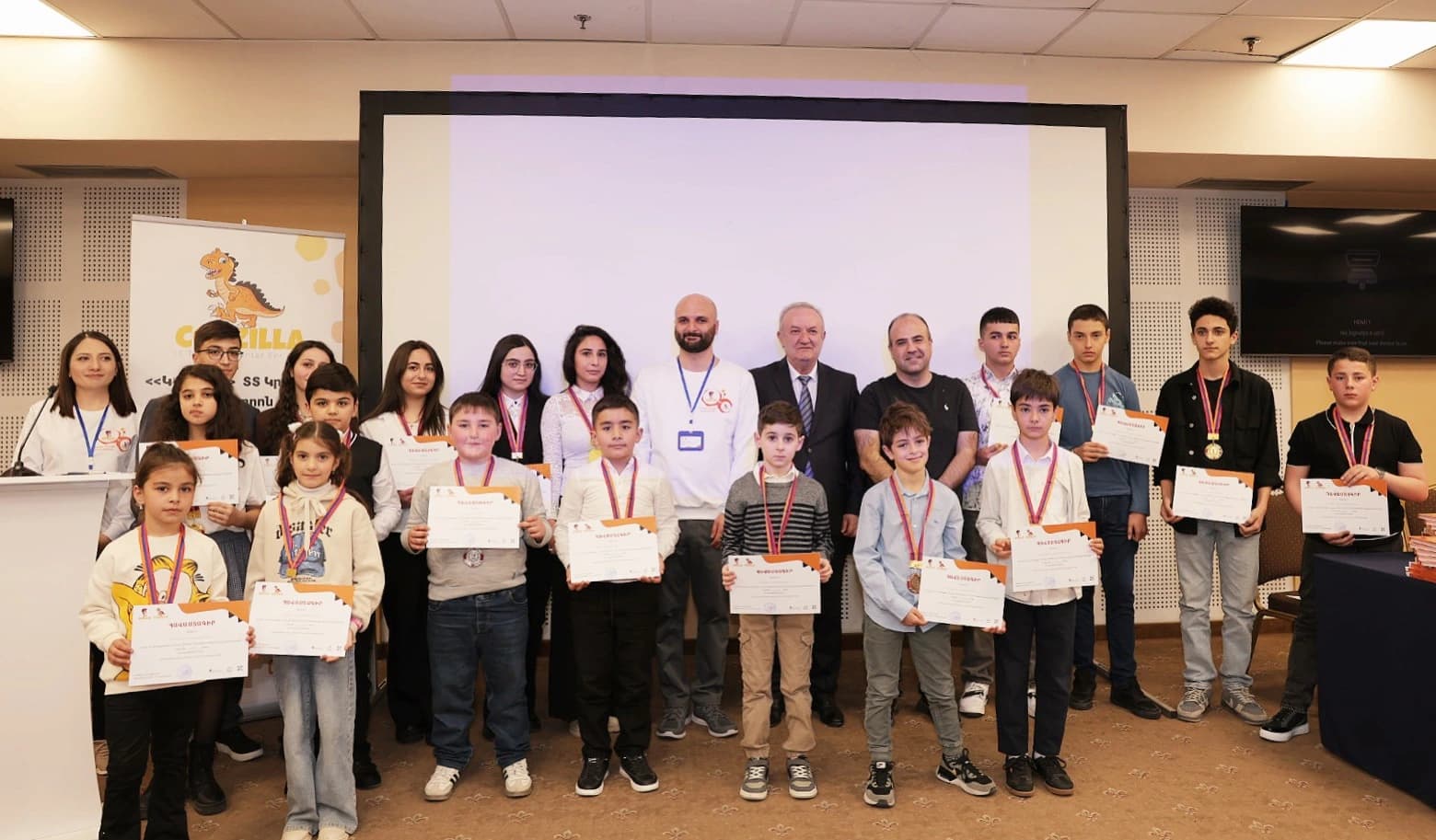
Patterns in administrative data can help schools optimize operations, from scheduling to resource allocation.
Efficient Resource Allocation
Analyzing patterns in resource usage, such as library book checkouts or computer lab usage, allows schools to allocate resources more effectively, ensuring that they are available when and where they are needed most.
📘 Tip: Use data visualization tools to monitor resource usage patterns and make informed decisions about future investments and allocations.
Optimizing Schedules
By examining attendance and performance data, schools can identify optimal times for classes and extracurricular activities, enhancing student participation and overall satisfaction.
💡 Insight: Flexible scheduling based on data-driven patterns can reduce conflicts and maximize the effectiveness of both teaching and learning activities.
✍️ Example:
If data indicates that students perform better in the morning than in the afternoon, schools might adjust their schedules to allocate more challenging subjects to the earlier part of the day, improving academic performance and student well-being.
Facilitating Data-Driven Decision Making
Data-driven decision making involves using data patterns to guide choices at every level of education, from classroom management to policy formulation.
Informed Curriculum Development
Analyzing patterns in student performance and industry trends can help educators design curricula that are relevant, up-to-date, and aligned with future career opportunities.
📘 Tip: Regularly review and update the curriculum based on data insights to ensure it meets the evolving needs of students and the job market.
Evidence-Based Policies
School administrators can use data patterns to develop and implement policies that address identified issues, such as attendance policies or support programs for underperforming students.
💡 Insight: Policies grounded in data are more likely to be effective because they are based on actual needs and proven strategies.
✍️ Example:
If data shows that students are more likely to drop out in their final year, administrators can develop targeted support programs, such as mentorship or counseling, to help retain these students and improve graduation rates.
Key Takeaways
- Enhancing teaching methods through pattern analysis helps in identifying learning gaps and tailoring instruction to individual student needs.
- Personalizing learning using adaptive systems and predictive analytics can significantly improve student engagement and success.
- Streamlining administrative tasks by analyzing usage patterns optimizes resource allocation and scheduling, making school operations more efficient.
- Data-driven decision making empowers educators and administrators to develop informed curricula and effective policies based on actual data insights.
🔍 Fun Fact: Personalized learning approaches, bolstered by data analysis, have been shown to increase student motivation and academic achievement by providing a more engaging and relevant educational experience.
Try This!
Self-Reflection Prompt:
Think about a recent teaching or learning experience. Can you identify any patterns in the data that could help improve future outcomes? How might you apply these insights to enhance your educational environment?
Conclusion
As we reach the end of our exploration into hidden patterns in data, it's clear that these invisible threads weave through every aspect of our educational landscape, influencing how we teach, learn, and manage resources. By uncovering and understanding these patterns, we equip ourselves with the tools to create more effective, personalized, and data-driven educational experiences.
Imagine a classroom where every action is informed by data—where teaching methods are continuously refined based on student performance, where learning is tailored to individual needs, and where administrative tasks are streamlined for maximum efficiency. This is the power of discovering hidden patterns in data. It's not just about numbers and charts; it's about enhancing human potential and fostering an environment where every student can thrive.
But the journey doesn't stop here. As technology advances and data becomes even more integral to our lives, the ability to recognize and interpret patterns will only grow in importance. For teachers and students alike, embracing these skills means staying ahead in a rapidly evolving digital world, ready to tackle new challenges with confidence and creativity.
So here's a thought-provoking question for you: How will you harness the power of hidden data patterns to transform your teaching or learning experience? Whether you're starting small by analyzing classroom data or diving deep into machine learning projects, the possibilities are endless. Embrace the challenge, explore the patterns, and watch as the hidden become revealed—turning data into actionable insights that make a real difference.
Empower Digital Minds Through Bebras
1,400 Schools
Enable every school in Armenia to participate in Bebras, transforming informatics education from a subject into an exciting journey of discovery.
380,000 Students
Give every student the chance to develop crucial computational thinking skills through Bebras challenges, preparing them for success in our digital world.
Help us bring the exciting world of computational thinking to every Armenian school through the Bebras Competition. Your support doesn't just fund a contest - it ignites curiosity in informatics and builds problem-solving skills that last a lifetime.
I Want to Donate Now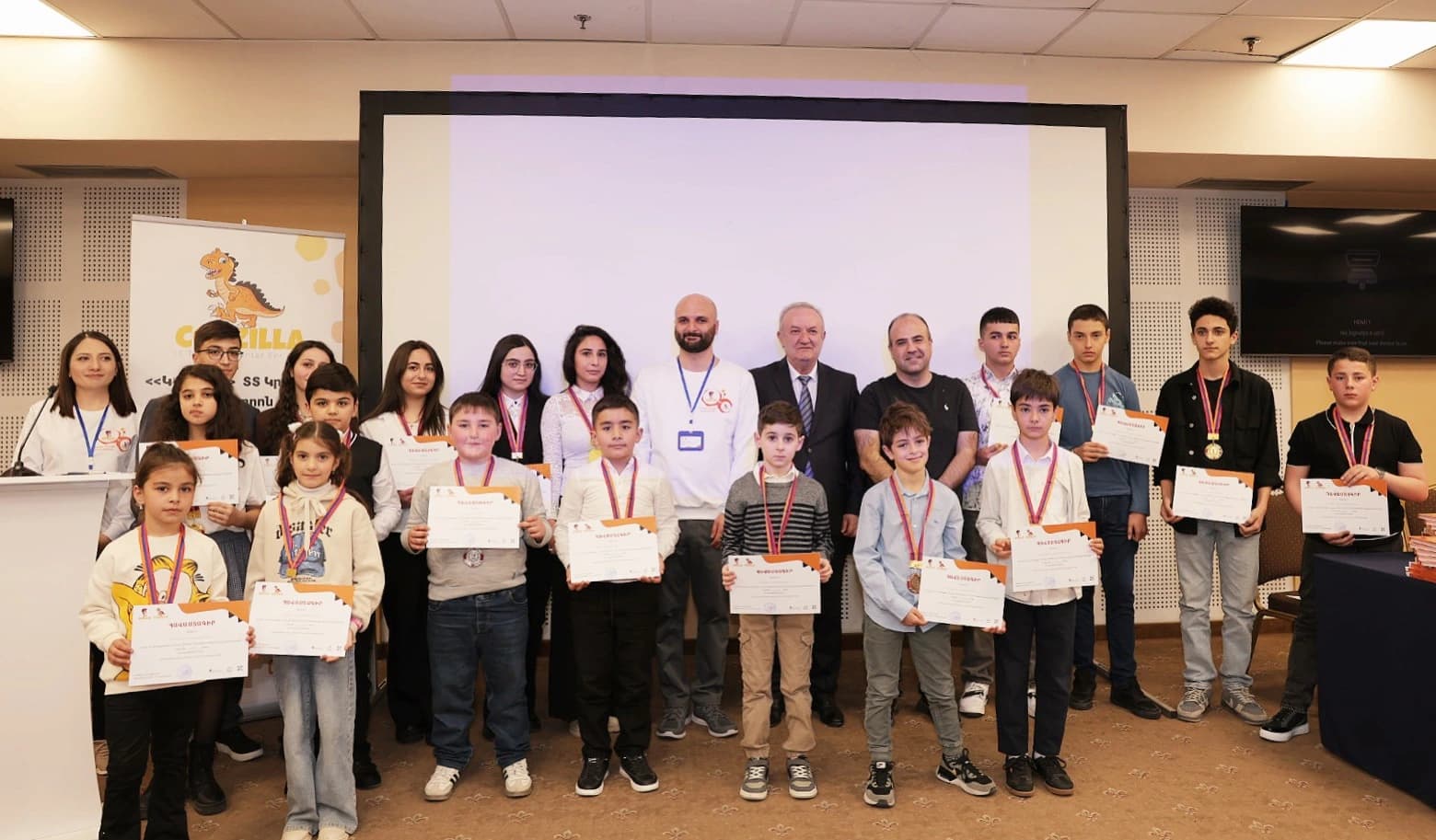
Final Takeaway
Hidden patterns in data are more than just academic concepts—they are powerful tools that can revolutionize education. By understanding and applying these patterns, teachers and students can create more effective, personalized, and engaging learning environments. So, let's embrace the data, uncover the patterns, and shape the future of education together!
Want to Learn More?
- Khan Academy: Data Analysis
- Coursera: Data Science Specialization
- Tableau Learning Resources
- Introduction to R Programming
- Scikit-learn Documentation
Key Takeaways
- Hidden patterns in data provide valuable insights that can enhance teaching methods, personalize learning, and optimize administrative tasks.
- Mastering data collection, cleaning, exploratory analysis, and machine learning techniques is essential for uncovering these patterns.
- Utilizing the right tools and technologies can streamline the process of discovering and applying data patterns in an educational setting.
- Embracing data-driven decision making leads to more effective and responsive educational environments, benefiting both teachers and students.
💡 Final Insight: The ability to discover and interpret hidden patterns in data is a skill that empowers educators and learners alike, fostering a more informed and adaptable educational experience.